Analytics in Genomics: Algorithms defining effective genomic research
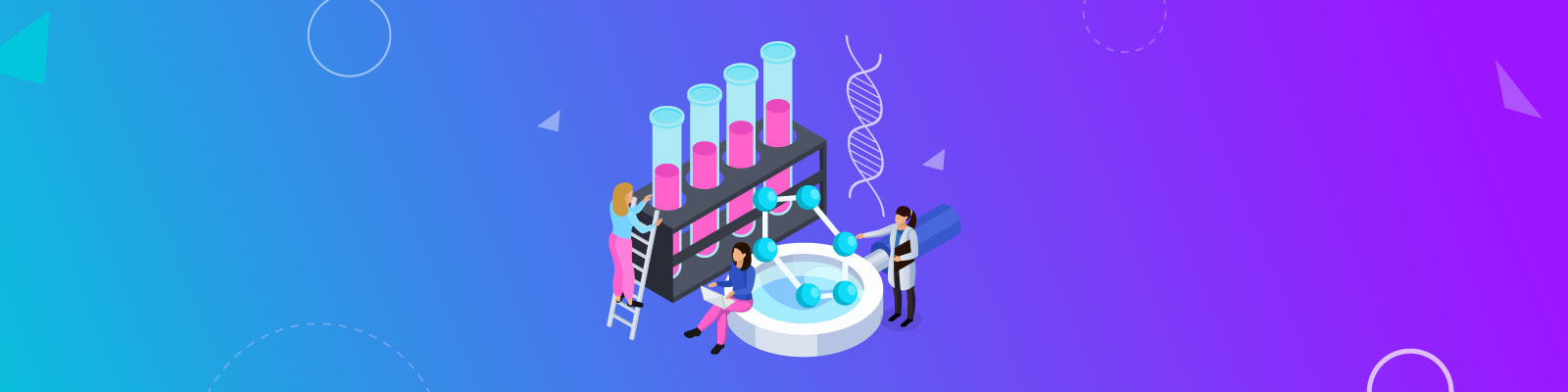
The genomic research processes were focused on limited data, until recently. In the last few decades, advances in genomic sequencing have facilitated the research processes with plethora of evolutionary data. In the arena of human genomics, it has fundamentally challenged the way diagnosis and medicine are being practiced. Today, healthcare and wellness decisions are becoming more personalized, using this genomic data. This has caused a paradigm shift in approaching diagnosis and healthcare in general. Human genome data has changed the way we think about the biological research processes today. In a traditional healthcare research organization, diagnosis has been primarily focused on the data related to a particular instant of the patient or the subject under study. In a way, this provides a snapshot of data for conducting the research, only for certain instants. Genomic research, on the other hand, helps in tracing the whole evolutionary journey until that point of time. This gives research a holistic aspect from an evolutionary perspective. In other words, the genomic data will have more biomarkers than before, which certainly enhances the quality of the allied research processes. However, the real value of these genomic datasets still remains unexplored to a large extent. Data analytics can potentially guide effective genomic research. The useful insights extracted from the genomic data can help in directing the problems to be solved in the research. For example, a normal research process would have the researcher search, store and curate information about each parameter in the research individually. This has been a traditional approach to conduct genomic research. Now, consider a data driven approach to conduct genomic research based on artificial intelligence (AI) methods. Here, the patterns defined by genomic data aid in modeling statistical inferences for prioritizing research processes. These statistical models form the basis of defining the AI algorithms. In other words, augmenting artificial intelligence with genomic data helps in generating useful insights. These AI algorithms and techniques help in deciding which of these research processes are useful. This cuts down the research time and enhances the research process quality exponentially.
The idea of employing artificial intelligence to conduct genomic analysis helps in generating better results, as compared to its manual counterpart. Considering the volume and precision required for genomic analysis, manual research processes are prone to subjectivity, limited scale and errors. AI algorithms learn from the patterns of genomic data. They leverage these patterns computationally and generate insights. These insights are at a massive scale, more precise and unbiased. This can dramatically improve the quality of genomic research processes. It is projected that the volume as well as the scale of the human genomic data is going to increase exponentially soon, as the cost of genome sequencing decreases. Inevitably, computational approaches, coupled with AI algorithms will redefine the genomic research processes in the near future.
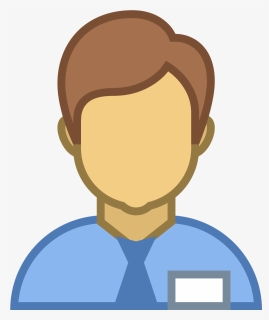
The Gene Box